Accelerating Preclinical Research with AI-Powered Literature Search
Preclinical in vivo work is the critical link between early-stage discovery and human clinical trials. This means it faces immense pressure to accurately demonstrate the potential of promising new therapies and to ensure their evaluation in models that reliably reflect human applicability. To achieve this, researchers need extensive knowledge of the available literature, especially recent advances, of which there is a growing number1–3. This underscores the importance of conducting effective and efficient literature searches to advance preclinical study goals and optimize preclinical design.
Traditional literature review methods are slow and tedious, and even with a massive time investment, they will never capture the full breadth of the available information. New AI solutions, like the ModernVivo platform, are streamlining literature reviews and offering profound insights in a fraction of the time.
This article will cover the centrality of effective literature searches in preclinical research, how AI solves perennial problems with traditional methods, and how researchers can overcome barriers to implementing AI in preclinical research.
The Critical Role of Literature Searches in Preclinical Research
Literature searches are crucial in preclinical research, enabling researchers to identify gold standards for in vivo study design and synthesize information from diverse sources4. However, traditional methods are riddled with inefficiencies that delay research timelines.
Why Literature Search Matters
Literature searches inform researchers about previous research and its methodology. This means they gain insights that direct hypothesis generation and study design, and facilitate alignment with regulatory requirements. Furthermore, they enable researchers to identify innovative approaches to address their research questions and pinpoint specific parameters to optimize their study design.
Common Bottlenecks in Traditional Methods
While literature databases contain essential information for guiding study design, this information is typically scattered across multiple papers and can be challenging to find. The fragmentation of information across different papers, disciplines, and databases severely limits the functionality of manual searches and makes exhaustive searches impossible. These limitations ultimately lead to inefficiencies and information gaps in experimental design.

How AI Enhances Literature Search for Preclinical Research
AI is driving improvements across the biomedical landscape, empowering more rapid and accurate diagnostics and streamlining research efforts5. Literature searches are an emerging area where AI is already having a transformative impact.
Automation and Intelligence
AI enables automated literature searches, meaning researchers can scan the entire body of biomedical literature for relevant information in seconds. AI allows interrogation of literature databases that moves beyond keyword searching and into the semantic understanding of the content, giving preclinical researchers more relevant information to help answer their specific research questions. Furthermore, AI can filter specific information from vast quantities of text, such as experimental conditions, species, and endpoints, saving researchers weeks or even months of time.
Advanced Capabilities
AI prioritizes studies that are most relevant to the researcher's query, meaning that they don’t need to read through abstracts or methods sections only to realize that the paper is irrelevant or outdated. Modern research is a multidisciplinary endeavor, meaning crucial information for in vivo experiments is fragmented across various disciplines. AI tools interrogate multiple fields instantaneously, generating an enriched pool of data and methodologies.
Overcoming Challenges in AI Integration for Preclinical Literature Review
Despite initial hesitation and skepticism, AI is now widely accepted as an integral part of the modern research landscape6. Failure to adopt AI-driven solutions puts researchers at a disadvantage and can threaten research success.
Beyond the Hype
Many early proponents of AI promised too much and failed to deliver tangible results7. While this disparity is becoming less frequent, it has understandably left many of us skeptical about adopting AI. That said, it is undeniable that AI has had a revolutionary impact on certain fields. For instance, AI can outperform trained radiologists in recognizing cancer in imaging tests5. Therefore, it is vital to embrace AI where it has demonstrated value while remaining cautious about areas where its progress has been limited, rather than adopting an all-or-nothing stance.
Ensuring Accuracy and Trust
Many researchers are concerned about a lack of oversight into how AI performs different tasks. This is a valid concern and is an area of ongoing research8. However, as AI becomes more mainstream, efforts to ensure its reliability and to validate its output are becoming more robust. As with many innovations, resistance to adopting AI can stem from a lack of understanding about what it is and how it works. It is important to recognize that AI is a tool intended to support, not replace, human efforts, and researchers can address their concerns by actively learning about its capabilities.
Managing Scientist Behavioral Shifts
As AI becomes more established, clinging to traditional workflows will lead to inefficiencies and, ultimately, hinder the success of preclinical research. Researchers can seek further education on AI by engaging with suppliers, attending conferences, and talking to peers who have successfully implemented AI tools in their workflows.
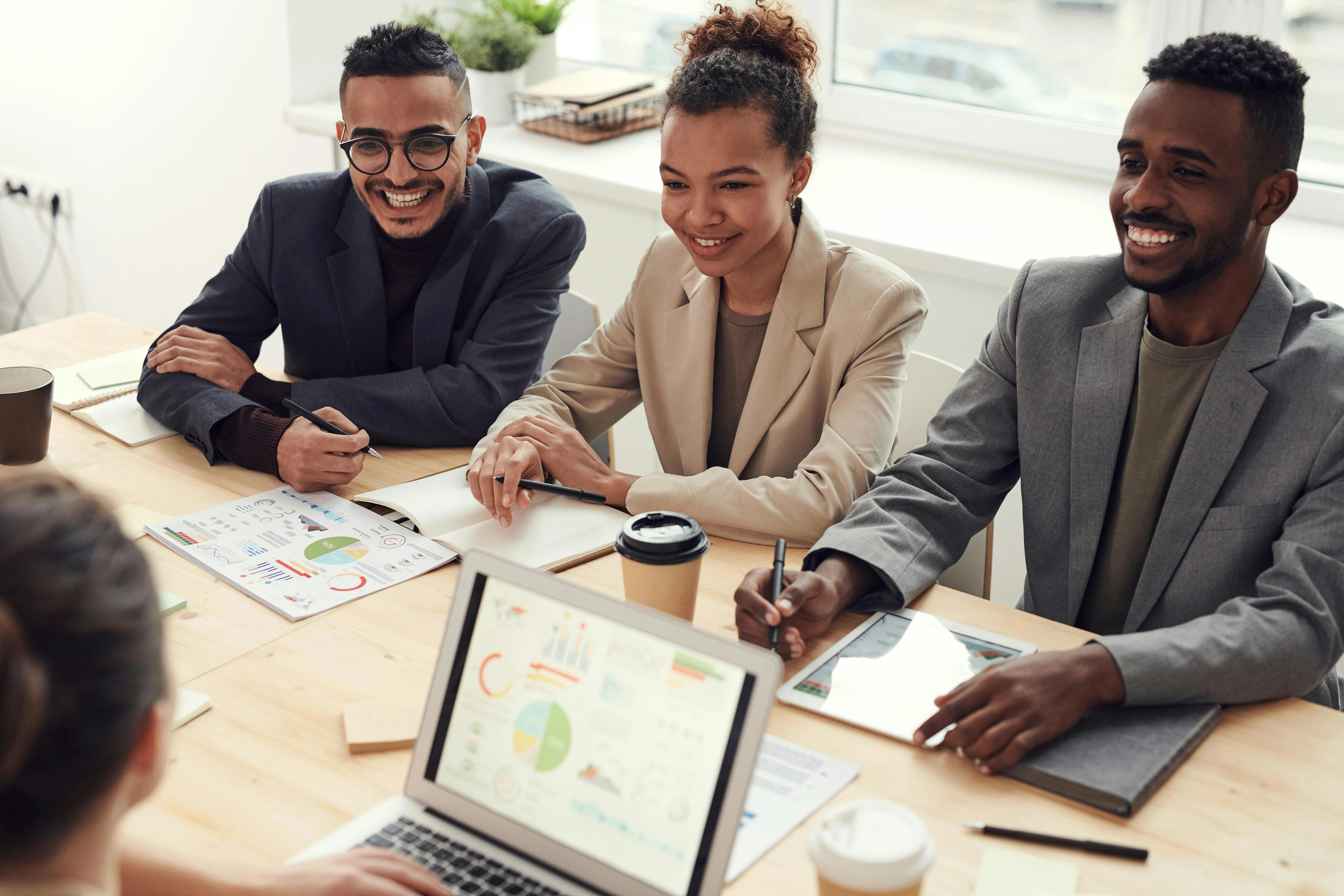
The Benefits of ModernVivo’s AI-Powered Literature Review Platform
ModernVivo has emerged as a leader in providing AI-powered literature reviews, empowering preclinical researchers to confidently tackle ambitious projects.
Key Features That Set ModernVivo Apart
ModernVivo uses semantic searches to derive deeper insights that better suit researchers' needs and far outperform the vague approach of keyword searches. By integrating multiple databases, ModernVivo provides a unified platform for interrogating the available literature, ensuring that researchers never miss out on important information that can help guide their preclinical study design. The platform is simple to use, meaning researchers can start accelerating their research with minimal training.
Advantages for Preclinical R&D
By adopting AI through the ModernVivo platform, researchers can instantly gain advantages in key areas:
- Speed: ModernVivo allows researchers to scan multiple databases with a single click, cutting the time required for literature collection and analysis from months to moments.
- Precision: ModernVivo generates hyper-specific results, eliminating duplicates and irrelevant data that often delay research timelines and are typical of manual methods.
- Cost-Efficiency: By minimizing the time required for literature searches, ModernVivo enables significant time and cost savings.
- Collaboration: ModernVivo was built for collaborative teams and facilitates team annotations, information sharing, and streamlined workflows.
The potential of AI to transform biomedical research is already being realized in literature searches and beyond. AI can benefit forward-thinking researchers in other areas, such as data collection and analysis, and predictive modeling9,10.
Ready to see how ModernVivo can transform your preclinical research workflows? Schedule a demo today!
FAQ
- How does AI-powered literature search differ from traditional keyword search methods?
AI-powered searches use semantic searches to achieve a deeper understanding of the available data, giving researchers only the most relevant information to guide their study design.
- What types of AI technologies are used in literature search tools for preclinical research?
Modern literature search tools use various AI technologies, such as natural language processing, machine learning, and ontology integration, to provide fast and accurate literature reviews.
- How reliable and accurate are ModernVivo’s AI-generated results in finding relevant preclinical studies?
ModernVivo users report a 75% reduction in study design development time for gold-standard approaches. This highlights how the platform gives researchers accurate, reliable, and actionable insights in a fraction of the time of manual methods.
References
1. Yadav R, Mahajan S, Singh H, et al. Emerging In Vitro and In Vivo Models: Hope for the Better Understanding of Cancer Progression and Treatment. Advanced Biology. 2024;8(6):2300487. doi:10.1002/adbi.202300487
2. Katti A, Vega-Pérez A, Foronda M, et al. Generation of precision preclinical cancer models using regulated in vivo base editing. Nat Biotechnol. 2024;42(3):437-447. doi:10.1038/s41587-023-01900-x
3. Sailer V, Von Amsberg G, Duensing S, et al. Experimental in vitro, ex vivo and in vivo models in prostate cancer research. Nat Rev Urol. 2023;20(3):158-178. doi:10.1038/s41585-022-00677-z
4. Ineichen BV, Held U, Salanti G, Macleod MR, Wever KE. Systematic review and meta-analysis of preclinical studies. Nat Rev Methods Primers. 2024;4(1):72. doi:10.1038/s43586-024-00347-x
5. Killock D. AI outperforms radiologists in mammographic screening. Nat Rev Clin Oncol. 2020;17(3):134-134. doi:10.1038/s41571-020-0329-7
6. AI and science: what 1,600 researchers think. Accessed December 13, 2024. https://www.nature.com/articles/d41586-023-02980-0
7. AI problems and promises | McKinsey. Accessed December 13, 2024. https://www.mckinsey.com/featured-insights/artificial-intelligence/the-promise-and-challenge-of-the-age-of-artificial-intelligence
8. Xu H, Shuttleworth KMJ. Medical artificial intelligence and the black box problem: a view based on the ethical principle of “do no harm.” Intelligent Medicine. 2024;4(1):52-57. doi:10.1016/j.imed.2023.08.001
9. Gao S, Fang A, Huang Y, et al. Empowering biomedical discovery with AI agents. Cell. 2024;187(22):6125-6151. doi:10.1016/j.cell.2024.09.022
10. Embedding AI in biology. Nat Methods. 2024;21(8):1365-1366. doi:10.1038/s41592-024-02391-7
AI Disclosure: Some of this content was generated with assistance from AI tools for copywriting. Images were generated by an AI image tool.