Designing in vivo experiments is fraught with challenges, from ethical considerations on wasted animal model studies to reliance on time-consuming manual literature reviews. These traditional methods often lead to suboptimal protocols and inconclusive results. ModernVivo, an AI-driven platform, revolutionizes in vivo study design by automating literature analysis, providing targeted insights, and enhancing both efficiency and compliance. This white paper highlights how researchers leverage ModernVivo to reduce in vivo study development time by up to 75%, improve protocol relevance, and meet evolving ethical standards in biomedical research.
In Vivo Experimental Design: Challenges and Imperatives
A well-designed study is essential for the success of any experiment. However, in vivo experiments carry extra considerations and risks that necessitate more attention to the details. At a fundamental level, in vivo studies involve the use of animals and carry a significant ethical dimension that is missing from in vitro work. Furthermore, they are far more costly to perform than routine in vitro experiments. While cost is less of a concern for larger laboratories with more budget flexibility to conduct repeat experiments, the stakes are much higher for smaller laboratories. Given all these factors, getting the details right from the start is crucial when performing in vivo work.
Limitations of Manual Literature Reviews in In Vivo Study Design
The most common approach to in vivo study design has not changed significantly in the last three decades. It’s probably not an exaggeration to say that the advent of the internet marked the last leap forward in efficient and accurate in vivo study design. For most researchers, interrogating the PubMed and Google Scholar databases with keywords remains standard practice. A small percentage of these users will use boolean operators, and an even smaller percentage will use them effectively. Even with a strong understanding of these tools, users can still struggle to find relevant papers to guide their in vivo study strategy.
With traditional approaches, users typically spend a month gathering information but only draw insights from 10-30 papers. Considering the abundance of information from decades of reported in vivo studies, this approach substantially underrepresents the available methodologies. Even in cases where researchers believe they have found what they are looking for, they are still leaving a lot to chance by only relying on a narrow collection of papers.
Many institutions continue to use protocols “handed down” from previous lab members. While using tried-and-tested protocols is generally a safe bet, researchers often miss out on novel approaches that may be more suitable to their needs. This is particularly true in today’s rapidly evolving research environment. New techniques, models, and assays are constantly emerging, requiring researchers to remain vigilant in evaluating the latest literature1. While routine techniques like western blot and PCR may benefit from long-standing protocols that have gradually become established within laboratory infrastructure, in vivo work must constantly evolve to match changing ethical, experimental, and regulatory standards. The bottom line is that traditional approaches lead to suboptimal in vivo experimental design and weeks or months of wasted time.
Common Pitfalls in Traditional Experimental Study Design
Traditional methods present several challenges for achieving optimal study design and allocating time and resources within research institutions.
- Risk of human error: Manual reading and transcription of study protocols can lead to misunderstandings and the generation of suboptimal protocols. Spending long hours reviewing materials and methods sections and cited papers to understand the science behind specific models can lead to reader fatigue and increase the likelihood of errors. Misinterpretations of seemingly minor details within an in vivo experiment can significantly affect study success. It is challenging to predict the effect of these parameters on study success until it is too late.
- Risk of human bias: All scientists are trained within a research environment that makes them prone to certain biases. Researchers might avoid implementing or considering certain protocols if they perceive the source as low-quality or untrustworthy. Furthermore, researchers tend to stick to what they know and may be unwilling to implement new models. This can lead to the selection of more expensive and less appropriate models.
- Inherent limitations of manual review: Manual literature reviews are inherently limited. We’ve already discussed how researchers rarely draw from more than 30 papers when designing a protocol, but in addition to a lower total number of papers, researchers typically draw these papers from the first few pages of literature database searches. This bias is inherent in manual literature review methods and can be particularly misleading as researchers feel they are somehow getting the “best” or “most appropriate” results where this may not be the case. In fact, lower-ranked papers often offer valuable insights that are missing from more polished, higher-impact papers, which may showcase impressive results but rarely include the troubleshooting and detailed decision-making involved in in vivo research.
- Reproducibility Concerns: In recent years, issues with reproducibility in in vivo research have surfaced across various biomedical disciplines, raising concerns about protocol accuracy and consistency. The complexity of animal models and their inherent variability often contribute to inconclusive or irreproducible results. Robust literature review leveraging the power of AI offers a novel approach to addressing these challenges by optimizing experimental design from the ground up.
Collectively, these challenges slow down the decision-making process for experimental design, reduce study quality, and can contribute to reproducibility problems within biomedical research2.
The Role of AI in Literature Review and In Vivo Experimental Design to Address Key Challenges
AI is improving how many tasks are performed within research and medical care. Profound advances have already been made in medical applications such as diagnosis and patient management3,4. Within drug discovery, AI is used for high-throughput screening experiments5, and in human clinical trials for participant selection and enrollment, trial design, and data collection, management, and analysis6. Given the advances elsewhere, the lack of AI-driven approaches for in vivo experimental design constitutes a significant bottleneck in the drug discovery pipeline.
AI significantly improves literature reviews by implementing natural language processing (NLP) and machine learning. This gives researchers a more refined yet global review of the available literature. It automates tasks like summarizing papers, identifying trends, and recommending important research, saving time and reducing the need for tedious and repetitive tasks. By leveraging AI, researchers can overcome limitations of traditional literature reviews, as highlighted below:
Timescale:
- Manual literature reviews take weeks to months to complete.
- AI-enabled literature reviews are completed in seconds to minutes.
Relevance and Validity:
- Manual processes are prone to bias.
- AI-driven methods are data-driven, providing more objective results.
Innovation:
- Manual reviews are resistant to changes and new technologies.
- AI-enabled approaches present the best options by leveraging advanced technologies.
Enhancing in vivo Study Design Efficiency
One of the key advantages of AI is its ability to accelerate workflows, which applies to nearly all AI applications, including literature reviews. AI can analyze and parse thousands of papers in seconds, which would take researchers weeks and months to achieve. Furthermore, AI is not prone to fatigue or biases that can disrupt human efficiency in these areas. Even with AI implementation, researchers will still need to manually review the highlighted studies to confirm they meet their needs. AI helps by directing their time and attention to the papers most relevant to their requirements, allowing them to begin experiments sooner and with greater confidence.
Manually reviewing the entire corpus of biomedical literature is impossible because it is already incredibly vast and is constantly growing. However, there is often significant redundancy in research, with many papers either using identical protocols or simply citing the original study instead of describing the methods. AI helps researchers save time by avoiding unnecessary citation chains and papers that provide no new insights.
Improved in vivo Study Relevance and Validity
AI helps researchers to pinpoint the most relevant information to apply to their studies. This involves providing researchers with high-quality studies that align with the current gold standards in research. Researchers often struggle to find papers that have what they need and may end up settling for papers that are ranked highly in databases but may not match their specific research focus.
Improving study validity and relevance with AI-driven tools has the obvious benefit of increasing the chances of study success but also comes with other advantages. In many cases, the correct design improves the work's credibility in the eyes of relevant stakeholders, such as reviewers, investors, and regulators, before the experiment has even been performed. Furthermore, well-designed and executed research will earn researchers reputational benefits when presenting data at conferences and through higher citation counts once their study is published.
Innovative in vivo Study Designs Enabled by AI
Most scientists are trained to take a rigid approach to study design. While this has advantages, it can blind them to more innovative approaches to addressing research questions. AI can analyze thousands of papers and suggest ways to apply different techniques that initially appear counterintuitive. This can lead to significant time savings and more efficient allocation of resources. Innovations need not be wholly transformative and may be as simple (yet important) as reducing the number of animals required to achieve statistical significance, predicting when a phenotype is more likely to emerge, and helping researchers optimize scheduling and research allocation more effectively. AI can be particularly useful for helping researchers adhere to the 3Rs of animal use in laboratory experiments7.
Furthermore, giving researchers insights beyond 1% of the available data may expose them to approaches they were unaware of, giving them a fresh perspective on the research area and thus increasing the chances for human-led innovation. AI helps researchers develop new strategies that are either highly innovative or simply optimize pre-existing protocols for efficiency and resource allocation.
Overcoming AI Bias and Regulatory Challenges in Literature Review
Although AI solutions can effectively reduce human errors and biases in literature review searches, they are still susceptible to introducing bias themselves. The methods used to train AI and the data it learns from can introduce bias in how the literature databases are interpreted and presented.
Sources of Bias in AI Systems
Many types of bias can prevent the optimal function of AI-driven literature reviews8. Below are two common examples:
- Data bias: This type of bias occurs when the dataset used to train the AI is incomplete or skewed, resulting in a representation that doesn't accurately reflect the broader global data. Thus, when the AI is applied to a real-world dataset, it will return less reliable results. This bias can arise from the continued appearance of an outdated protocol for a specific research question, one that was widely used in the past but has since been replaced by a more modern and effective model. AI might misinterpret the overrepresentation of this method in the dataset as evidence that it is the most appropriate approach.
- Algorithmic bias: This occurs when the algorithm used by the AI to search through literature databases is biased towards reporting specific subsets of data and avoiding others. While all algorithms will have some biases, it’s essential to understand where these biases are beneficial and where they lead to poorer outcomes. For instance, algorithms that rank publications based solely on citations may miss out on more modern (though less cited) research. AI algorithms may also rely on simplified keywords to quickly process large amounts of information. This can be problematic in complex datasets where specific niche details are important. Additionally, such biases can inadvertently reinforce existing disparities in academic research, perpetuating underrepresentation of particular approaches or models.
Solutions for Bias in AI Systems
AI systems require constant updating and appraisals to ensure they remain relevant and suitable for their intended use. Working out and resolving biases in AI systems is a continuous process.
- AI Auditing: This is the process of continuously evaluating AI systems, including the data used for their training and the algorithms they use, for suitability and adherence to ethical and regulatory requirements. Auditing is vital for identifying and correcting biases in areas where AI-informed decisions can have significant real-world implications, such as in vivo study design, drug discovery, and healthcare more generally9.
- User Insight: It is crucial for AI systems to constantly learn from users so that they can be turned more accurately to their needs. Expert scientific insights are fantastic for identifying where AI accurately identifies the gold standard for answering specific research questions. Ideally, the AI will be developed by a team that combines user-specific insights with the expertise of impartial AI specialists.
Ethical Considerations
One central area where AI systems struggle to adhere to ethical standards is transparency10.
This is often referred to as the "black box problem," where users input a command, the AI runs an algorithm that the user doesn’t understand, and the generated results are used to inform decision-making. This creates ethical issues as researchers and associated stakeholders, such as regulators and patients, cannot see the decision-making process. This raises problems with consent, data security, and accountability. In the latter case, AI complicates the assignment of responsibility for any harm caused by AI-driven decisions. The concept of explainable AI (XAI) has emerged to help address this challenge11.
AI promotes the ethical treatment of animals in in vivo studies by optimizing study designs to ensure that only the minimum number of animals necessary are used to achieve the study's objectives. In many cases, this can lead to a reduction in the number of animals used, directly contributing to the 3Rs (Replacement, Reduction, Refinement) and promoting animal welfare7. ModernVivo supports compliance with Institutional Animal Care and Use Committee (IACUC) protocols, minimizing ethical concerns with the use of animal subjects.
Regulatory Compliance
Regulatory requirements have been explicitly designed to counteract complex ethical issues raised by AI. Much of this is focused on increasing the transparency of AI-driven decisions but also aims to safeguard sensitive data. Users often provide AI with sensitive data, which raises issues with privacy and consent. A prominent example of this is patient health data; however, scientists using AI for literature review reveal information about their research goals, intellectual properties, and what reagents they will likely use in the near future. Thus, AI must be tightly regulated to handle user information carefully and securely.
Ultimately, AI is a tool intended to assist, not replace, human efforts. As in other areas of drug discovery and healthcare, combining human input and AI-driven tools for in vivo study design will likely lead to better outcomes than either alone12. Human oversight remains crucial for addressing AI's blind spots, mitigating potential biases, and ensuring adherence to ethical standards.
AI-Powered Experimental Design: How ModernVivo Transforms In Vivo Studies
ModernVivo provides the latest AI-driven solutions for in vivo study design. It provides researchers with the most relevant studies for their work, eliminating the need to search through extensive databases manually. ModernVivo significantly de-risks and speeds up in vivo design by offering researchers only the essential, gold-standard parameters necessary to improve their chances of success.
Without ModernVivo, in vivo researchers have two options: manual literature reviews, which are inherently long and prone to error and bias, and non-specific AI tools, which are not trained to provide optimized information for in vivo work. Researchers who use poorly designed AI tools struggle to cite their rationale for choosing specific parameters and have less control over how their data is used.
ModernVivo overcomes common limitations to manual methods and existing generic AI solutions. Below are two real-world case studies where ModernVivo has streamlined researchers' goals for designing optimal in vivo work.
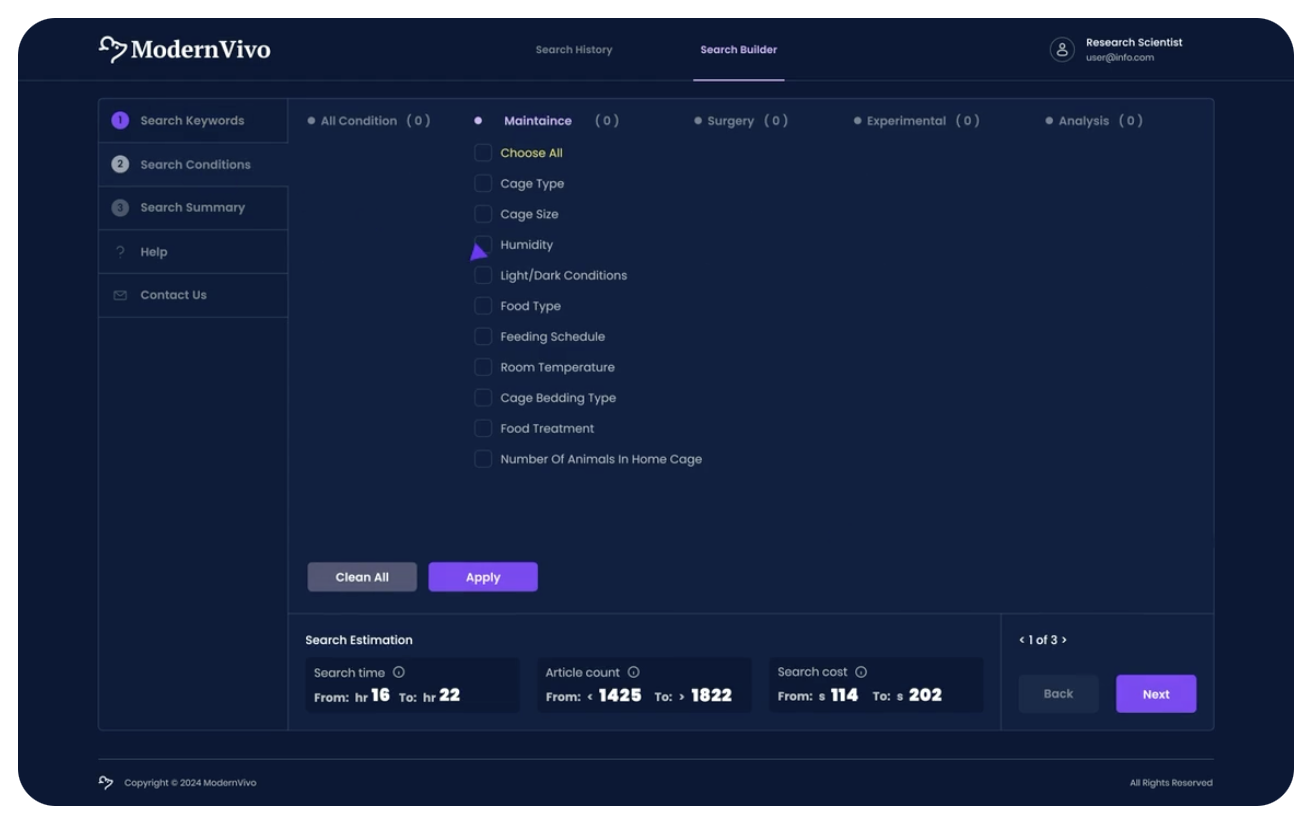
Case Study #1: Achieving a 75% Reduction in Experimental Development Time for Accelerated Study Execution and Cost Efficiency
Pancreatic Ductal Adenocarcinoma Research Group
The Problem: Pancreatic cancer is a complex and deadly disease with very high mortality rates due to being asymptomatic until the later stages13. Studying pancreatic cancer is also challenging for many reasons. The in vivo studies available to study pancreatic cancer are incredibly diverse, and different assays are only suitable for answering specific research questions. Given these challenges, the client struggled to identify ideal experimental conditions and faced significant delays in their study design timeline.
Client Request: The client approached ModernVivo with the goal of analyzing all existing literature for in vivo models of pancreatic cancer to aid in the identification of the ideal animal model and study design parameters.
Solution: The client used the ModernVivo platform to analyze design methods from 1271 academic papers in a matter of hours.
Results: The client achieved a 75% reduction in experimental development time, leading to accelerated study execution, improved design confidence, and significantly reduced costs.
Case Study #2: Reducing Study Design Time from One Month to One Hour with Streamlined Protocol Development
Alcohol Addiction Research Group
The Problem: Alcohol addiction is a multifactorial disease, and there is a plethora of diverse animal models available to study it14. However, the abundance of methods and the volume of existing literature make it challenging to hone in on a precise set of parameters for a given study. Thus, the client found it impossible to manually review the literature while adhering to their project timeline.
Client Request: The client approached ModernVivo with the goal of conducting a comprehensive literature review to design a behavioral study focused on alcohol addiction. They wanted to develop a gold-standard methodology for their study, incorporating established research practices and recent advancements.
Solution: The client used the ModernVivo platform to analyze ~3500 published papers.
Results: ModernVivo helped the team create an appropriate study protocol in just one hour, significantly shortening their usual study design process, which typically took about a month.
Want to Learn How ModernVivo Significantly Reduces In Vivo Experimental Design Time?
The integration of AI-driven platforms like ModernVivo is revolutionizing in vivo experimental design. Traditional literature review methods are slow, error-prone, and limited in scope. ModernVivo enables researchers to quickly analyze thousands of relevant studies, reducing biases and improving the validity of their research. Streamlining the design process significantly reduces costs and provides researchers with a robust, ethical, and often innovative approach to in vivo research. As AI evolves, tools like ModernVivo will be essential for designing in vivo research and improving outcomes in an increasingly complex scientific landscape.
ModernVivo is available for research teams across academia and industry to use immediately. We are committed to a fair and balanced pricing system, aiming to support the next generation of in vivo experiments by ensuring researchers only pay for what they actually need.
Visit our webpage or contact a member of our team to try the ModernVivo platform today.
Not sure if ModernVivo is the solution you need? Schedule a demo today to see the power of this revolutionary technology for yourself.
References
1. Yadav R, Mahajan S, Singh H, et al. Emerging In Vitro and In Vivo Models: Hope for the Better Understanding of Cancer Progression and Treatment. Advanced Biology. 2024;8(6):2300487. doi:10.1002/adbi.202300487
2. Gannot G, Cutting M, Fischer D, Hsu L. Reproducibility and transparency in biomedical sciences. Oral Diseases. 2017;23(7):813-816. doi:10.1111/odi.12588
3. Alowais SA, Alghamdi SS, Alsuhebany N, et al. Revolutionizing healthcare: the role of artificial intelligence in clinical practice. BMC Med Educ. 2023;23(1):689. doi:10.1186/s12909-023-04698-z
4. Bi WL, Hosny A, Schabath MB, et al. Artificial intelligence in cancer imaging: Clinical challenges and applications. CA A Cancer J Clinicians. 2019;69(2):127-157. doi:10.3322/caac.21552
5. The Atomwise AIMS Program, Wallach I, Bernard D, et al. AI is a viable alternative to high throughput screening: a 318-target study. Sci Rep. 2024;14(1):7526. doi:10.1038/s41598-024-54655-z
6. Hutson M. How AI is being used to accelerate clinical trials. Nature. 2024;627(8003):S2-S5. doi:10.1038/d41586-024-00753-x
7. The 3Rs | NC3Rs. Accessed October 21, 2024. https://nc3rs.org.uk/who-we-are/3rs
8. Vicente L, Matute H. Humans inherit artificial intelligence biases. Sci Rep. 2023;13(1):15737. doi:10.1038/s41598-023-42384-8
9. Mökander J. Auditing of AI: Legal, Ethical and Technical Approaches. DISO. 2023;2(3):49. doi:10.1007/s44206-023-00074-y
10. Quinn TP, Jacobs S, Senadeera M, Le V, Coghlan S. The three ghosts of medical AI: Can the black-box present deliver? Artificial Intelligence in Medicine. 2022;124:102158. doi:10.1016/j.artmed.2021.102158
11. A. S, R. S. A systematic review of Explainable Artificial Intelligence models and applications: Recent developments and future trends. Decision Analytics Journal. 2023;7:100230. doi:10.1016/j.dajour.2023.100230
12. Ellis R, Ellestad E, Elicker B, Hope MD, Tosun D. Impact of hybrid supervision approaches on the performance of artificial intelligence for the classification of chest radiographs. Computers in Biology and Medicine. 2020;120:103699. doi:10.1016/j.compbiomed.2020.103699
13. Kleeff J, Korc M, Apte M, et al. Pancreatic cancer. Nat Rev Dis Primers. 2016;2:16022. doi:10.1038/nrdp.2016.22
14. Moussas G, Christodoulou C, Douzenis A. A short review on the aetiology and pathophysiology of alcoholism. Ann Gen Psychiatry. 2009;8(1):10. doi:10.1186/1744-859X-8-10
"WT and TK rat photo" by Jason Snyder is licensed under CC BY 2.0.
AI Disclosure: Some of this content was generated with assistance from AI tools for copywriting.