Balancing Efficiency and Ethical Integrity in Preclinical Research
Drug development has always been a lengthy and challenging process. However, modern development teams face growing pressure to accelerate drug discovery pipelines1. To meet these demands, technological advancements such as AI, in silico modeling, organoid-based in vitro research, and laboratory automation have emerged. Despite these innovations, speed must not come at the expense of ethical and regulatory frameworks that ensure safety, efficacy, and public trust in new treatments. Researchers must strike a balance between efficiency and adherence to these critical standards. This blog explores best practices for responsibly optimizing preclinical workflows, ensuring that acceleration does not compromise ethical and scientific integrity.
Ethical Imperatives in Preclinical Research
Numerous ethical and regulatory frameworks ensure that research prioritizes safety, animal welfare, ethical integrity, and patient well-being. These include:
- IACUC - Institutional Animal Care and Use Committees (IACUC) ensure ethical treatment and welfare of animals in research, which can be difficult to adhere to without the proper considerations2.
- The 3Rs - Replace, Reduce, and Refine principles guide researchers in minimizing animal use while maximizing ethical standards3.
- FDA and EMA - The U.S. Food and Drug Administration (FDA) and the European Medicines Agency (EMA) enforce rigorous regulations to ensure drug safety and efficacy4.
Reproducibility and transparency are fundamental ethical pillars that modern research must uphold and strengthen through improved practices5. This includes designing robust, well-thought-out studies and maintaining full transparency and honesty when reporting and sharing data. Pre-competitive collaboration and data standardization are key to enhancing research integrity by promoting openness, reducing bias, and improving efficiency6. By adopting standardized data and methodologies, researchers can accelerate discoveries and ensure the reliability of scientific findings.
Strategies for Ethical and Efficient Preclinical Research Acceleration
Several strategies have emerged in recent years that allow researchers to drive faster drug discovery pipelines while adhering to regulations and ethical integrity standards.
- Automation enhances the speed and accuracy of various drug discovery workflows, particularly high-throughput screening assays7.
- Adaptive clinical trials enable flexible trial designs that adjust based on emerging data, improving efficiency and reducing delays while maintaining rigor8,9.
- Fast-track programs, including Breakthrough Therapy and Accelerated Approval, speed up approval for treatments targeting serious conditions10.
- Data-sharing initiatives between academia, industry, and regulators enhance research efficiency by reducing duplication and promoting innovation11,12.
Let's delve into some advancements that are helping to streamline research and drive compliance in more detail.
AI and In-Silico Models for Enhanced Preclinical Study Design
AI is significantly accelerating various aspects of drug discovery. Predictive analytics enable researchers to integrate vast datasets, including genomics and pharmacokinetics, to refine drug candidate selection while reducing the need for resource- and time-intensive testing13. These analytical tools also support predictive toxicity assessments and the identification of potential mechanisms of action. Digital twin technology—virtual models of biological systems—offers a powerful tool for simulating drug responses, further optimizing preclinical research14.
Rather than completely replacing traditional, ethically aligned methodologies, AI primarily enhances and accelerates existing approaches. Nonetheless, ethical and regulatory standards are evolving to accommodate AI’s expanding role in preclinical studies15,16.
Advancements in In Vitro Models to Complement Traditional Approaches
In vitro models are increasingly complementing in vivo approaches in preclinical studies, allowing researchers to utilize advanced methods while conducting more ethically responsible experiments. Notable innovations include organoid models and automated high-throughput screening techniques. Organoids, composed of diverse cell types, more accurately replicate the structure and function of human tissues than cell lines cultured in 2D, improving the study of drug responses, disease progression, and toxicity17. Integrating these advanced models into high-throughput screening allows researchers to test multiple compounds simultaneously, significantly expanding both the quantity and depth of data18. This approach also aligns with regulatory and ethical standards while potentially reducing the reliance on animal testing in preclinical research19.
Optimizing In Vivo Study Design for Reproducibility and Efficiency
Modern research applications that prioritize efficiency and accuracy are pushing researchers to evaluate their study designs more critically. This involves formulating well-defined hypotheses, which facilitate more targeted decision-making when selecting appropriate models. Additionally, researchers are increasingly working to reduce bias by implementing improved randomization and blinding techniques in animal studies20,21. These strategies help refine optimal approaches to enhance reproducibility. Moreover, by integrating the principles of the 3Rs, researchers can improve study rigor while maintaining higher animal welfare standards3.
Leveraging Real-World Data for Preclinical Optimization
Modern preclinical research benefits from access to a vast body of literature and established study designs, which help guide research efforts. This allows researchers to avoid redundant experiments, select the most appropriate models for specific research questions, and make more data-driven decisions. Additionally, leveraging existing data supports study design rationale and helps minimize the use of animals in cases where similar questions or models have already been explored.
The Future of Ethical Preclinical Research Acceleration
AI is becoming increasingly central in this field by enabling the rapid analysis of large datasets and literature databases, leading to more ethical and efficient preclinical study optimization. By supporting evidence-based research practices, AI allows researchers to select optimal models without the need for redundant experiments or multiple rounds of optimization. This accelerates research while ensuring alignment with ethical standards for animal welfare.
As the field evolves, researchers must critically evaluate their reasons for not adopting AI while also ensuring its use complies with guidelines and maintains necessary human oversight in preclinical studies. In response to the growing role of AI, the FDA released a guidance document in January 2025 to help researchers integrate AI responsibly while remaining compliant16.
Collaboration across technology, life sciences, and regulatory bodies like the FDA is essential to advancing research efficiently while leveraging the full potential of AI-driven innovations11,12.
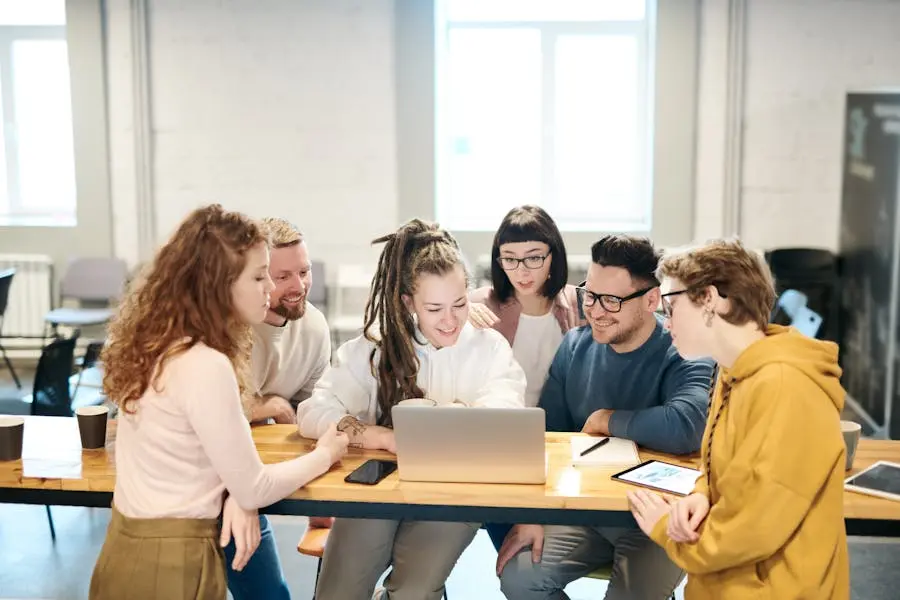
ModernVivo: Advancing Preclinical Research Without Compromise
ModernVivo is equipping scientists with cutting-edge AI-driven tools for in vivo assay design, enabling them to harness recent advancements while maintaining strong regulatory and ethical alignment. Effective research leverages past experiments and modern technology to uphold ethical integrity while meeting the demands of modern drug discovery.
Companies that adopt platforms like ModernVivo can seamlessly integrate state-of-the-art technology with insights from previous research, positioning themselves for regulatory approval and long-term success.
Schedule a demo!
Get in touch with the ModernVivo team today and book a demo to see how you can improve the efficiency, compliance, and ethical rigor of your preclinical research.
References
1. Deloitte pharma study: R&D returns are improving | Deloitte Switzerland. Accessed March 5, 2025. https://www.deloitte.com/ch/en/about/press-room/deloitte-pharma-study-r-and-d-returns-are-improving.html
2. The IACUC. https://olaw.nih.gov/resources/tutorial/iacuc.htm
3. The 3Rs | NC3Rs. Accessed March 5, 2025. https://nc3rs.org.uk/who-we-are/3rs
4. Langhof H, Chin WWL, Wieschowski S, Federico C, Kimmelman J, Strech D. Preclinical efficacy in therapeutic area guidelines from the U.S. Food and Drug Administration and the European Medicines Agency: a cross-sectional study. Br J Pharmacol. 2018;175(22):4229-4238. doi:10.1111/bph.14485
5. Armond ACV, Cobey KD, Moher D. Research Integrity definitions and challenges. Journal of Clinical Epidemiology. 2024;171:111367. doi:10.1016/j.jclinepi.2024.111367
6. Thibault RT, Amaral OB, Argolo F, Bandrowski AE, Davidson AR, Drude NI. Open Science 2.0: Towards a truly collaborative research ecosystem. PLoS Biol. 2023;21(10):e3002362. doi:10.1371/journal.pbio.3002362
7. Holland I, Davies JA. Automation in the Life Science Research Laboratory. Front Bioeng Biotechnol. 2020;8(571777). doi:10.3389/fbioe.2020.571777
8. Pallmann P, Bedding AW, Choodari-Oskooei B, et al. Adaptive designs in clinical trials: why use them, and how to run and report them. BMC Med. 2018;16(1):29. doi:10.1186/s12916-018-1017-7
9. Research C for DE and. Adaptive Design Clinical Trials for Drugs and Biologics Guidance for Industry. April 21, 2020. Accessed March 5, 2025. https://www.fda.gov/regulatory-information/search-fda-guidance-documents/adaptive-design-clinical-trials-drugs-and-biologics-guidance-industry
10. Commissioner O of the. Fast Track. FDA. Published online August 13, 2024. Accessed March 5, 2025. https://www.fda.gov/patients/fast-track-breakthrough-therapy-accelerated-approval-priority-review/fast-track
11. Hulsen T. Sharing Is Caring-Data Sharing Initiatives in Healthcare. Int J Environ Res Public Health. 2020;17(9):3046. doi:10.3390/ijerph17093046
12. Karpen SR, White JK, Mullin AP, et al. Effective Data Sharing as a Conduit for Advancing Medical Product Development. Ther Innov Regul Sci. 2021;55(3):591-600. doi:10.1007/s43441-020-00255-8
13. Vora LK, Gholap AD, Jetha K, Thakur RRS, Solanki HK, Chavda VP. Artificial Intelligence in Pharmaceutical Technology and Drug Delivery Design. Pharmaceutics. 2023;15(7):1916. doi:10.3390/pharmaceutics15071916
14. Hernandez-Boussard T, Macklin P, Greenspan EJ, et al. Digital twins for predictive oncology will be a paradigm shift for precision cancer care. Nat Med. 2021;27(12):2065-2066. doi:10.1038/s41591-021-01558-5
15. Chopra H, Annu null, Shin DK, et al. Revolutionizing clinical trials: the role of AI in accelerating medical breakthroughs. Int J Surg. 2023;109(12):4211-4220. doi:10.1097/JS9.0000000000000705
16. Research C for DE and. Considerations for the Use of Artificial Intelligence To Support Regulatory Decision-Making for Drug and Biological Products. July 1, 2025. Accessed March 5, 2025. https://www.fda.gov/regulatory-information/search-fda-guidance-documents/considerations-use-artificial-intelligence-support-regulatory-decision-making-drug-and-biological
17. Kim J, Koo BK, Knoblich JA. Human organoids: model systems for human biology and medicine. Nat Rev Mol Cell Biol. 2020;21(10):571-584. doi:10.1038/s41580-020-0259-3
18. Shinozawa T, Kimura M, Cai Y, et al. High-Fidelity Drug-Induced Liver Injury Screen Using Human Pluripotent Stem Cell-Derived Organoids. Gastroenterology. 2021;160(3):831-846.e10. doi:10.1053/j.gastro.2020.10.002
19. Park G, Rim YA, Sohn Y, Nam Y, Ju JH. Replacing Animal Testing with Stem Cell-Organoids : Advantages and Limitations. Stem Cell Rev Rep. 2024;20(6):1375-1386. doi:10.1007/s12015-024-10723-5
20. Karp NA, Pearl EJ, Stringer EJ, Barkus C, Ulrichsen JC, Percie du Sert N. A qualitative study of the barriers to using blinding in in vivo experiments and suggestions for improvement. PLoS Biol. 2022;20(11):e3001873. doi:10.1371/journal.pbio.3001873
21. Verhave PS, van Eenige R, Tiebosch I. Methods for applying blinding and randomisation in animal experiments. Lab Anim. 2024;58(5):419-426. doi:10.1177/00236772241272991
AI Disclosure: Some of this content was generated with assistance from AI tools for copywriting.